Regola di automazione di Jira quando una pull request viene sottoposta a merge
Posted by: Sheridan Gaenger
The feeling you get when you first unlock the power of data is not unlike the feeling that Prince Adam gets when he turns into He-Man. Self-service business intelligence exists to provide this feeling to anyone who wants it by making data easy to use for everyone.
By “everyone,” we do mean everyone — from data wizards to the least technically inclined. Seth Rosen, co-founder of Hashpath, recently tweeted that using Atlassian Analytics as a self-service BI platform was one of the first times he “felt like [he] had super powers as a data analyst.”
Self-service BI is indispensable for any company that calls itself “data-driven,” thanks to its ability to make data accessible to all. If you’re in the market for a self-service BI platform, here’s what you need to know.
Self-service BI increases access to data
Self-service BI provides end business users (i.e., non-technical folks) with the ability to analyze data and create visualizations on their own without the help of technical teams. In contrast, traditional BI tends to require significant technical expertise to use, which can lead to a data bottleneck.
There is no clear line of delineation between what makes a business intelligence platform “self-service” or “traditional.” It’s best to think of it as a spectrum.
On one end, traditional BI platforms prioritize strict control over data. Only a few experts have access to the platform, let alone the technical know-how to use it well. As a result, these traditional platforms don’t have to invest in usability, as their main focus is empowering a select few to use data.
Here’s an example of creating a SQL query with Mode Analytics, a more traditional BI tool that focuses on the needs of data scientists:
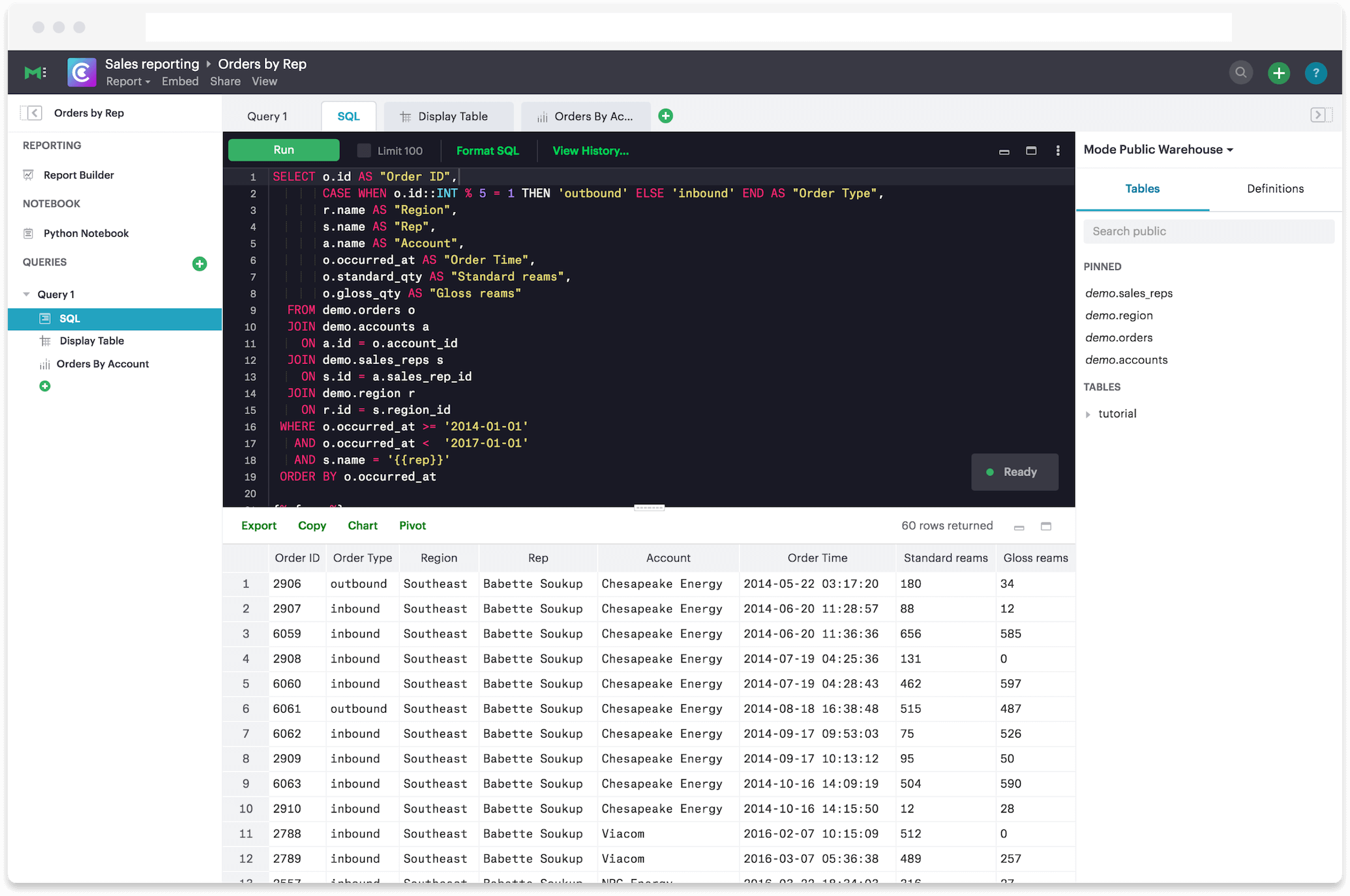
On the other end, self-service BI platforms prioritize access to data. These platforms focus on putting data at the fingertips of as many people as possible. As a result, self-service BI platforms invest heavily in slick user-experience design and no-code features.
When a self-service BI platform does its job well (i.e., providing access to data), the impact can be pretty huge. Let’s take the workflow of getting access to data, for example. The easier it is for anyone at your company to use a BI platform, the less they need to go through technical teams (like your data team, IT department, or your developers) to use it. In short, people with no technical background can explore data on their own.
This freedom to explore promotes ad hoc analysis, where you can go wherever the data takes you without a preset plan. Exploring on your own means that you won’t be bugging your technical team with one-off requests. You can find answers for yourself, and the technical team can focus on longer-term goals instead of fielding ad hoc requests.
Apply this freedom across the whole company, and you’ve got a bona fide data culture, where data becomes central to day-to-day decision-making.
Andare oltre Agile
Self-service BI has a reputation for relinquishing tight control in favor of wider access to data. The logic goes: if more people that can use data, the less control there is over how the data gets used. But good self-service tools provide both access and control to company data. And, all the while, it teaches its users how to use data well.
Technical teams are the only ones with the skill set to effectively use and manage traditional BI platforms. This position of power over data gives these teams tight control over how the company uses data. But this control comes at a cost — every request, issue, and query will have to go through the technical team.
A good self-service BI platform solves this bottleneck problem in three ways:
- Provides widespread access to data
- Maintains control over how data gets used
-
Helps bridge the data literacy gap
An example of a self-service BI platform helping companies maintain control over their data is Atlassian Analytics’s own ability to manage, create custom schemas, and access the full schema. A schema is a “collection of tables and their relationships in your database.”
Put simply, control over your schemas means control over the organization of your data. And control over data organization means control over how people access that data.
But control over who has access to what is just one piece of the puzzle. You need to take into account the “data literacy gap” that can come with self-service BI.
Your technical team is already data literate (they went to school for it), but your CMO, sales development reps, or any other end business users aren’t. Put another way, just because an end business user can explore and use data on their own with a self-service BI tool doesn’t mean they can explore and use data well.
If you put a self-service BI tool at the disposal of end business users, you also need to invest in the data literacy of these users. A good self-service BI tools will make developing data literacy a natural part of the workflow. It will nudge users in the right direction without overriding their goals.
In Atlassian Analytics’s case, as we were developing Visual SQL, we looked back at 10 years of query data and found that we could predict, with 88% accuracy, how our users wanted to aggregate and group their data. So now, Atlassian Analytics goes ahead and automatically groups the data in a way it thinks the user wants. Even if it predicts incorrectly, providing something the user can react to is not only useful but also educational because they can discover new ways of grouping they hadn’t considered.
We often find even the most non-technical users are bitten by the “data bug” sooner or later. These users often want to dive deeper to use data more effectively. To help in these cases, we developed the Data School to help bridge the gap in data literacy that self-service BI tools can sometimes create.
Andare oltre Agile
All BI tools have three main functions: gather, understand, and visualize data. To evaluate self-service BI tools, you need to dive a little deeper to understand their implementation, ease of use, and dashboarding features.
Quick implementation
A good self-service BI tool will be easy to implement and maintain. There are two indicators of easy implementation:
- The library of integrations. You want to ensure all your data sources (e.g., CRM, databases, data warehouse) are easy to connect to the BI platform using direct connections. If the BI tool doesn’t have a direct connection to the data source you need, you’ll have to do a custom connection, which will require your IT department to get involved.
- Time to first dashboard. Look at reviews and documentation for how long it takes to go from purchasing a license to creating your first dashboard. We have estimations for all the major BI tools here.
Ease of use
A self-service BI platform needs to be intuitive to use for both technical and non-technical folks. In particular, you should look at:
- How easy it is to connect a new data source. Connecting new data sources should be easy enough for end business users to do on their own without necessarily having to loop in a technical team member.
- If it’s possible to query data with no knowledge of SQL. Visual SQL query builders (like Visual SQL) allow you to build queries with little to no code.
- How intuitive the data visualization process is. It should be a matter of one or two clicks to turn your queried data into data visualizations and put them into dashboards.
Great dashboards
The final product of a good self-service BI platform is the dashboards it helps you to create. When evaluating the dashboarding capabilities, look for:
- Visualization variety. Intuitiveness is important in the process of creating visualizations, but you also need to consider the quality and variety of visualizations you can choose from.
- How easy it is to share dashboards. Also, look for a variety of ways to share your dashboards, from Slack alerts to embedding.
In-dashboard collaboration. Finally, widespread access to data should go hand-in-hand with in-dashboard collaboration.
Affordability
Self-service BI tools should be worth the money you invest in them. To avoid sunk costs, look for flexibility and avoid lock-in.
- Flexible pricing. Make sure they have flexible pricing plans that make it affordable for any size team (from one to hundreds) to use the platform.
Avoid lock-in. Your needs may change as your company grows, so make sure the platform doesn’t lock you in with long-term contracts.
Scalability
Purchasing a self-service BI tool should be an enduring investment that grows alongside your business. A few features that indicate a self-service BI tool that can scale well include:
- Custom tables and schemas: As you grow, the functionality you need out of your data grows, too. The ability to create custom tables and schemas provides flexibility to your data that allows it to adapt to your changing needs.
Data stores: A data store will help you control who gets access to specific types of data. For instance, you might create a data store for marketing initiatives, which contains every data point a marketing team member might need, without any excess. Data stores are indispensable as your company grows because they allow you to manage access to your data granularly.